There is a spectre haunting our lives, and that is chaos theory. It’s all around us, but we hardly see it. Understanding it gets to the heart of some of the most interesting debates and dichotomies in academia: humanities versus social science, inductive versus deductive reasoning, classical versus romantic, hippies versus yuppies.
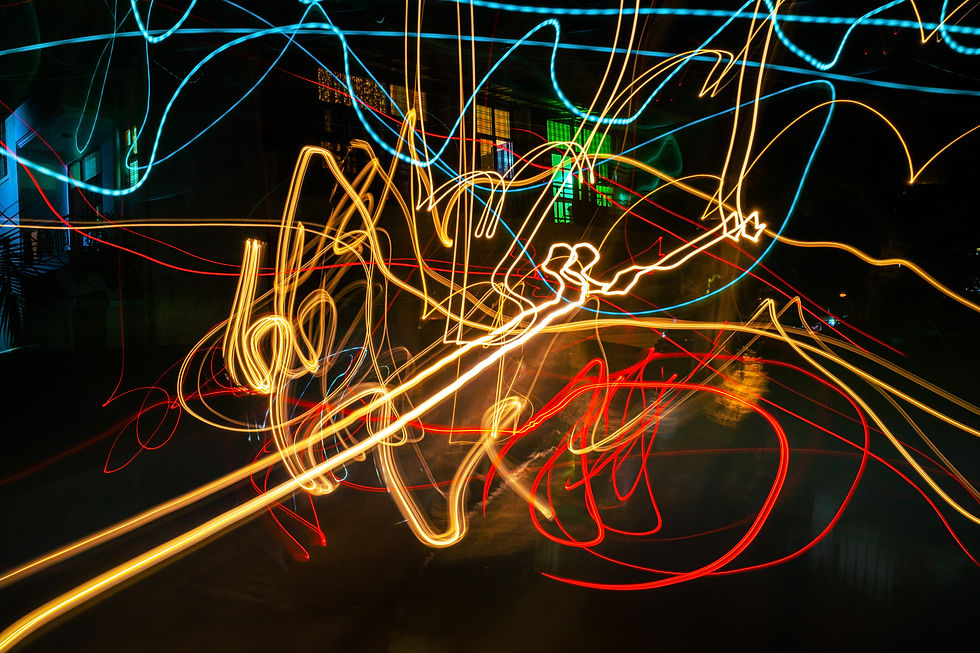
Picture by Saheb Zaidi, Unsplash
In the 17th century, Francis Bacon published the New Organon, ushering in the modern age of scientific discovery. We still struggle to know the true causes of things, but we’re a far way off from thinking the universe was made of 4 elements or defining humans as ‘featherless bipeds’ (cue Diogenes plucking the feathers off a chicken and chucking it into the forum, taunting Plato, ‘Behold, a man!’). But epistemologically, we have to ask ourselves how advanced we really are: modern medicine was mostly useless until the 1930s; we live in an age where quackery and misuse of statistics and information is ripe. This is because, as is relatively common knowledge now, humans often act quite irrationally. This simple truism, I posit, is partly why we have so much trouble not just predicting future actions, but even explaining past ones as well.
One way we can think about the limits of our knowledge is the application of ‘chaos theory’, which behind its cool name lies a deceptively powerful concept that most social scientists would do well to heed. At its heart, chaos theory argues ‘that some social systems are characterized by ‘chaotic’ behaviour which precludes the possibility of predicting the future of that system’. Most famously popularized by notions such as the ‘butterfly effect’, whereby even the smallest event (e.g. a butterfly flapping its wings over Cambridge) can have a massive impact (a hurricane over Kowloon).
This idea has its roots in another crucial development of statistics (themselves a pillar of any social science and prediction), namely ‘Laplace’s Demon’, which pondered whether if one knew the exact location and direction of every atom in the universe, could one predict the future? Chaos theory says no, for both practical and epistemological reasons. Why can’t we know the future?
Often it is a measurement problem. We can’t measure every atom, let alone to a degree that would allow us to predict their future. An example to highlight this is in weather forecasting, and it was when chaos theory was first ‘discovered’. Even in physical systems (with no sentient beings to model, who are fickle to say the least), minutia matters. In the computer-infancy 1980s, when Lorenz was modelling climate patterns and wanted to rerun an experiment through his computer, he manually reinputted his numbers into the machine. However, he only put in 8 decimal places (this is all the printer printed off for him). This change is infinitesimal – a millionth of a degree – but despite having changed no parameters, the lack of these miniscule decimals was enough to make the results completely different. How can a few decimal places affect the outcome so radically? In a nutshell, this is the effect of chaos on physical systems.
But how does this work in social science? Chaos in social systems has two key definitions:
It detectable by its ‘highly iterative, recursive, or dynamic structures that change over time’.
It demonstrates ‘highly discontinuous behaviour in the system’ – this is sometimes also described as being ‘fractal’.
One famous example of this are the so-called Mandelbrot Sets, in which for 'a substantial portion of the values of the [input], extremely close neighbours behave in opposite manners’. Or, in layman’s terms, Mandelbrot Sets are equations which demonstrate stability and instability in a pattern that is completely unpredictable: inputs so close and similar can generate wildly different outputs. This unpredictable relationship is the mathematical (and natural) manifestation of chaos. And this is why chaos theory is so important to social sciences: one can build complex models, with multivariate prediction algorithms – and end up completely wrong.
What else can be tried to remedy this? What about increasing the number of actors (‘increasing our n-value’, in the parlance of statisticians) – will this help us forecast with more certainty? Sadly, also no: ‘even in a study with a large number of actors, there can be a very large number of cases where arbitrarily similar actors can display radically different behaviours.’ Even our fanciest mathematical tricks – statistics, linear and non-linear regressions, or structural equations – are woefully unhelpful when studying chaotic systems.
So, do we just give up? Yes. And no.
It is true that ‘many social systems reflect the central components of chaos theory.’ Understanding chaos theory helps direct us towards where we can predict – and helps us avoid where we can’t. When studying chaotic systems (as described above), traditional social science often relies on a few worn-out tricks: a) searching (in vain) for some omitted variable that will explain the chaos, b) thinking their measurement is the problem, or c) worrying that the stochastic part has overwhelmed the patterned part (and not seeing that randomness is the pattern, essentially). Like the Bed of Procrustes, we should be wary of fitting chaotic systems onto models which rely on tools for studying non-chaotic systems.
This blog post does not claim to have the solution but knowing what we’re doing wrong – i.e. using cross-sectional research designs to fallaciously predict the chaotic world around us – is as good a start as any.
コメント