Electoral maps in the United States are re-drawn at a state level every ten years, based on a set of ambiguously defined criteria that include compactness of districts, competitiveness, geographical contiguity, and compliance with the Voting Rights Act (VRA). The partisan nature of many of the committees responsible for redistricting leads to decision making that often favors one party above the other; a practice that is called “gerrymandering”. As part of ongoing literature that aims to quantify and assess the fairness and level of gerrymandering present in state electoral maps, Gregory Herschlag et al's (2020) paper applies a Markov Chain Monte Carlo (MCMC) sampler[1] to build an ensemble of redistricting plans; current maps are tested against this ensemble to assess partisan gerrymandering.
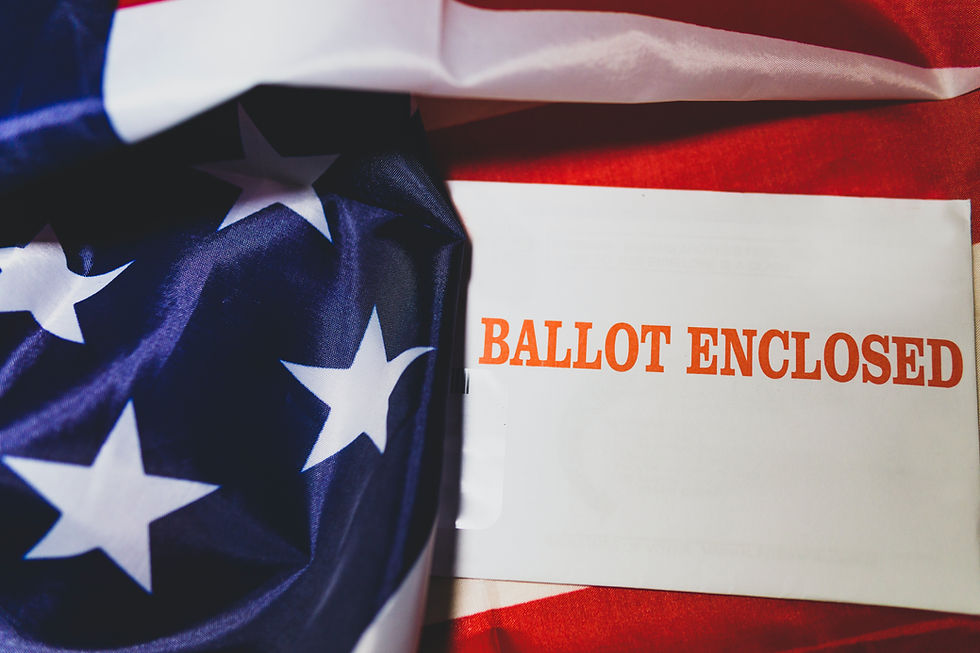
In simple terms, this paper aims to solve the following problem: we want to have an ideal, “fair” districting plan to which we can compare the current maps drawn by partisans. However, no such plan exists! Building an “ensemble” of plans means figuring out a process by which one can repeatedly draw maps that are created with the purpose of being “fair” under specific criteria. While the math is complex, the idea that Herschlag et al employ is quite intuitive: define a penalty function that can quantify which redistricting plans come closer to being “fair”, then apply the function alongside a mechanism that sequentially proposes new maps. This process is repeated until an ensemble is formed.
The benefit of having an ensemble is that it can be used to apply distribution level tests that compare the real district plans to those that are simulated by the MCMC process. In this paper, the authors compare three different US House maps from North Carolina (NC) to an ensemble built using a penalty function tuned to the requirements of the state constitution and the VRA. They conclude that, across a variety of metrics, the two maps drawn by the NC legislature are extreme outliers. The punchline is that it is highly unlikely the maps have been drawn with the goal of fairness in mind.
While the conclusions drawn about NC congressional maps are both interesting to the lay reader and present potential for use in one of the many redistricting court cases that are currently being fought, the method is the core of the paper. Assuming that no flaws exist in the process, this tool simulates the perfect legislator, who redistricts with exclusively constitutional criteria. However, this turns out to be a relatively large ask. While the authors perform a long laundry list of tests to ensure the validity of the method, others have later pointed out flaws that may exist in the sampler; this is not a closed field of research, and other algorithms are constantly being developed.
Notes:
More about redistricting can be found on Professor Justin Levitt’s webpage, and a very accessible introduction to Gerrymandering is available from FiveThirtyEight's Gerrymandering Project
[1] A Markov Chain is a sequence of events that only depend on their exact previous state. Given an unknown distribution of outcomes to sample from, MCMC methods focus on defining a sequence (or “chain”) that approximately samples from that distribution, given it is run for enough timesteps.
Comments